Our research aims to develop computational approaches to gain biological or clinical insights into human disease. Using genetic variation as a foundation, in particular “high impact” variants from large-scale sequencing studies, we hope to shed insight into genes, disease processes, and experimental manipulations that could one day lead to improved treatments or patient care.
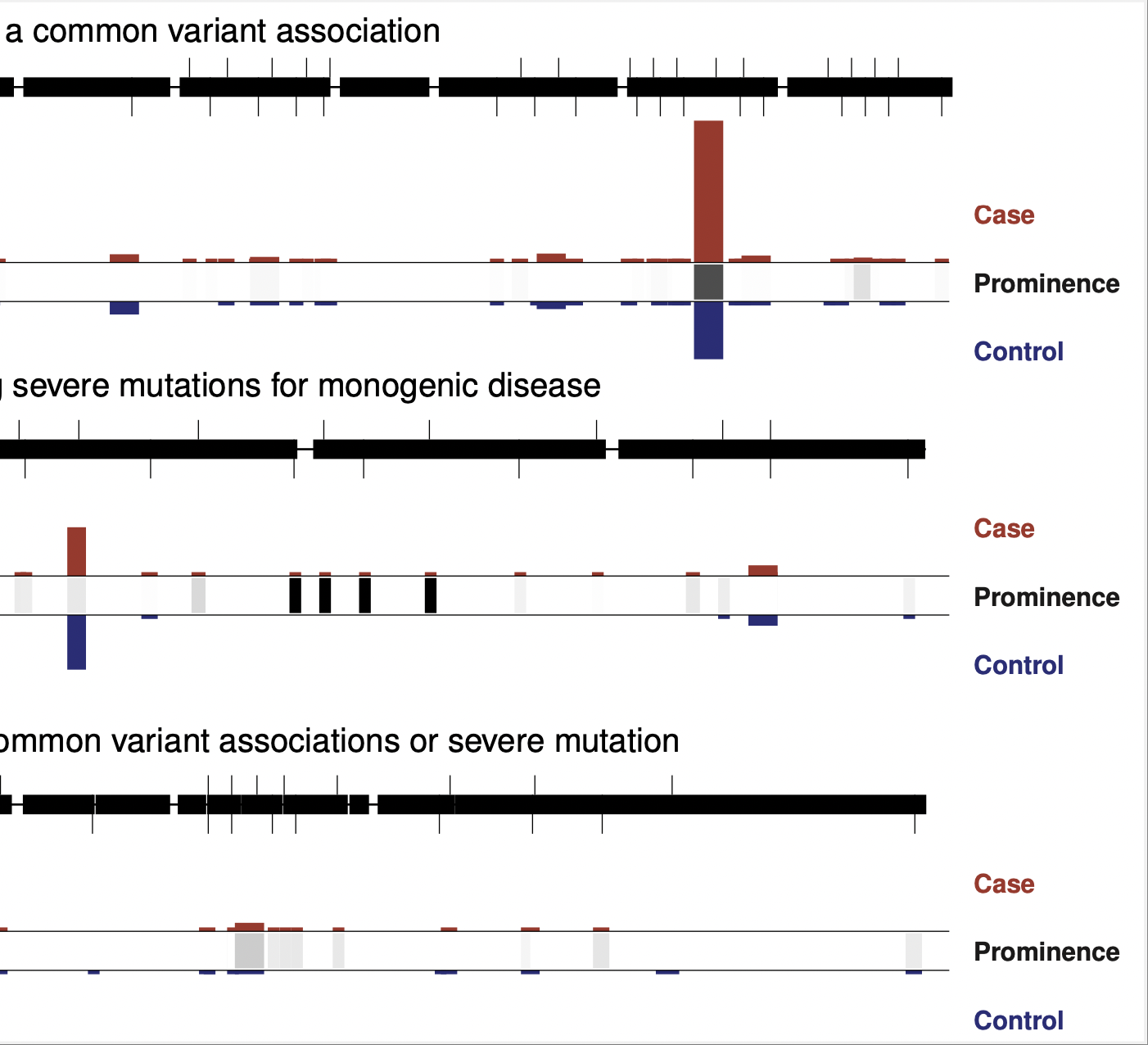
Rare variant association studies
Our lab grew from rare coding variant association studies, which began in earnest around 2009 as large-scale sequencing became cost-effective. Rare coding variants provide direct links between disease and a gene – arguably the most important goal of a genetic study – and therefore offer an extremely valuable complement to genome wide association studies, or GWAS, which do not immediately implicate genes in disease.
You can read about our prior work on T2D rare variant associations on our publications page, or see slides from a presentation on the history of T2D rare variant discovery if you would prefer a digest. We most recently quantified the role of coding variants in the genetic architecture of T2D and established their potential utility in genetic risk prediction.
Lab projects in this area are ideal starting points for prospective lab members with minimal experience in programming or statistics. There are lots of interesting analyses of large-scale sequencing data that can be done to help understand the role that rare coding variation plays in disease and the implications this has for future study designs.